
Greg Hager
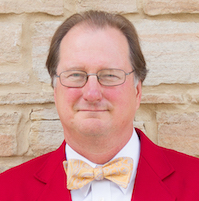
Mark Hill
The following is a guest blog post from CCC Vice Chair and Post Moore’s Law Computing Task Force Chair Mark Hill from University of Wisconsin-Madison and former CCC Chair and Artificial Intelligence Task Force Chair Greg Hager from Johns Hopkins University.
In a recent article, “Chips off the Old Block: Computers Are Taking Cues From Human Brains,” the New York Times highlighted the latest new wave of innovation in computer hardware, the foundation of Information Technology that has so altered our world. Like many generations of innovation before it, these innovations are being driven by the insatiable need for additional computing capacity, in this case due to the new demands of the latest generation of machine learning (ML) innovations.
However, this change also comes at a time when there is a broader fundamental shift in the growth of computing capacity. As background, mid-20th century computer hardware has repeatedly doubled in performance every two years or so through the synergistic interaction of technology improvements (Moore’s Law) and architectural innovations in how to effectively use the ballooning transistor budget. Until early in the 21st century these improvements were transparent to software developers except that applications ran much faster, enabling far richer applications. The latest advances have increasingly relied on the use of multicore chips and specialized hardware such as graphics processing units (GPUs). From the applications perspective, the improvements of the last decade have required changes in the software stack that are substantial, but still relatively evolutionary.
The New York Times article highlights the fact that the current revolution is demanding more radical changes to the boundary between software and hardware, especially for machine learning. As past experience with in other areas, such as high performance computing, have shown, it is not always easy to take advantage of new high performance hardware while supporting familiar, easy-to-use high-level languages and interfaces. Fortunately, the latest changes are still largely transparent to ML experts as they are in fact designed to support some of the high-level interfaces, such as Google’s TensorFlow, that are in wide use already. Systems highlighted by the article include Microsoft’s use of field-programmable gate arrays (FPGAs), Google’s tensor processing unit (TPU) to support TensorFlow, and Nvidia’s advances in GPUs.
For those interested, the CCC has sponsored several workshops and white papers related to these ideas dating back to 2012 including the 21st Century Computer Architecture white paper and several more-recent whitepapers from nanotechnology through architecture to cross-cutting and onto machine learning and brain connections.
At least the next decade of computer hardware metamorphosis promises to be exciting!