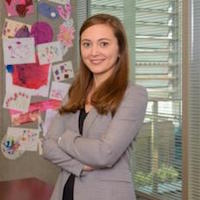
Nadya Bliss
The following blog post was contributed by Nadya Bliss (Director, the Global Security Initiative at Arizona State University & CCC Council Member) and is reposted from the Delta 8.7 website. You can view the original post here.
Advances in computational science and artificial intelligence offer opportunities to advance Target 8.7 of the Sustainable Development Goals, but the anti-trafficking community must first establish some core building blocks that can serve as the foundation upon which new technologies can be developed and shared. Simply throwing flashy new tech at the problem is neither strategic nor effective. Key components of this foundation include a shared strategy, a common infrastructure that allows for better and more sharing of data and a pipeline that shepherds ideas from basic research to applied research to operationalization and finally to demo and validation.
The session “Towards a Pipeline – Technology, Techniques and Training” tackled this challenge by looking at four opportunity areas that can be categorized into different components of the research pipeline:
- Is artificial intelligence a silver bullet? (basic research)
- Common data collection and taxonomy protocols (applied research)
- Data trusts (applied research)
- Getting the tech community involved (demo and validation)
Developing a pipeline is well-defined in other domains. A good example to draw from is the United States Department of Defence guidelines on science and technology research, which defines different components of the pipeline from basic research through implementation.
Artificial intelligence (AI) fits into the basic research or applied research component of this pipeline. Anjali Mazumder of the Turing Institute explained that it is not a silver bullet, but it does present opportunities if leveraged properly. Specifically, AI can help:
- Measure prevalence and map vulnerability;
- Prevent trafficking by helping to understand drivers and pathways to exploitation, and identify possible interventions;
- Pursue perpetrators and identify victims, and build decision support systems to better predict potential occurrences;
- Prosecute perpetrators by building tools that are reliable and admissible in court; and
- Support victims and the anti-trafficking community through more efficient resource allocation.
For AI to be effective though, tools must be developed with a recognition of the potential for algorithmic bias, with relevant legal frameworks in mind and by multidisciplinary teams that include non-technologists.
Data sharing is a key component of the operationalization of any tool in the area. This requires a shared infrastructure that promotes more and deeper collaboration, both among sectors (industry, academia, industry) and actors in the anti-trafficking community (NGOs, governments, research organizations).
The first step in this type of infrastructure is developing data standards: documented agreements on representation, format, definition, structuring, tagging, transmission, manipulation, use and management of data. Harry Cook of the International Organization for Migration discussed current efforts to create such data standards. One primary benefit of data standards is that it creates readable data that can be automatically shared and distributed among disparate actors. Developing a common language that allows different actors to use the same terminology with the same meanings will also help with transparency.
Challenges to creating data standards are significant, with the privacy of survivors paramount. Thus data standards must include rules for governance such as avoiding personally identifiable information (PII) whenever possible, establishing strong protections for PII if collected and ensuring survivors are able to provide input into how data is collected and used. Finally, data should not be collected blindly, simply to collect data. All data collection efforts should have a clear purpose.
Data trusts could be critical to effective data sharing, as explained by Steve Anning of the British Army. Data trusts are proven and trusted frameworks and agreements that ensure exchanges of data are secure and mutually beneficial.
The anti-trafficking community is multi-sector, multidisciplinary and international, and consequently has different ideas of how to handle data. Data trusts would allow collaboration as a community in a trusted manner, enhancing opportunities for successful analysis and effective policy-making.
There are three key components of a data trust:
- Shared ethics: members of the trust must use agreed-upon methodologies;
- Architectural: a portal for use of data by members;
- Governance: pre-agreed upon procedures for handling data.
Getting the tech community involved can foster the growth of capacity in front-line organizations and the groups working on the ground to support survivors and combat trafficking. Large tech companies have significant expertise building technology that works and deploying it widely and effectively, skills that can be beneficial to anti-trafficking efforts. Phil Bennett started Tech Against Trafficking to do just that, but noted two requirements for these efforts to be successful:
- Technologists must get involved in the space to avoid becoming distant from the human story and the potential consequences of their technology.
- Second, he cited the need to start building the pillars of infrastructure that are necessary for information sharing and increased collaboration.
This session focused on areas that align with the beginning and end stages of a research pipeline. The next steps need to focus on the middle stages, or operationalization, and how to translate these ideas and efforts into an executable plan. This will require identifying metrics that can be used to measure progress toward the goal.
This piece has been prepared as part of the Code 8.7 Conference Paper. Read all the contributions here.
This article has been prepared by Nadya Bliss as a contributor to Delta 8.7. As provided for in the Terms and Conditions of Use of Delta 8.7, the opinions expressed in this article are those of the author and do not necessarily reflect those of UNU or its partners.
Learn more about the CCC’s work with Code 8.7 on the blog and in the Catalyzing Computing podcast.