This blog post includes contributions from Josh Deutsch (UC Santa Cruz), Mike DeWeese (UC Berkeley), and Lee Altenberg (University of Hawaiʻi at Mānoa).
In early January, the Computing Community Consortium (CCC) hosted a visioning workshop on Thermodynamic Computing in Honolulu, Hawaii in order to establish a community of like-minded visionaries; craft a statement of research needs; and summarize the current state of understanding within this new area of computing.
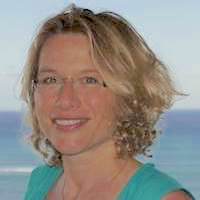
Susanne Still
Following the Thermodynamic Computing workshop, the CCC sponsored the related Manoa Mini-Symposium on Physics of Adaptive Computation at the University of Hawaiʻi at Mānoa. Susanne Still (University of Hawaiʻi) was one of the leaders of the Thermodynamic Computing workshop and organized the mini-symposium, which featured nine Thermodynamic Computing workshop participants as speakers. General attendees included faculty and grad students from the University of Hawaiʻi.
The speakers and the topics of their presentations were:
- Seth Lloyd, MIT – “Learning to make energy free” (related paper)
- Gavin Crooks – “Thermodynamic constraints of communication and interaction in coupled system”
- Rob Shaw, Haptek – “Computation with Shapes“
- Lee Altenberg, University of Hawaii Manoa- “How much information can natural selection maintain?“
- Chris Watkins, Royal Holloway – “A model of evolution that satisfies detailed balance“
- Massimiliano Esposito, University Luxembourg – “Thermodynamic cost of Information Processing“
- Josh Deutsch, UC Santa Cruz – “How to make a computer out of junk DNA“
- Lidia del Rio, ETH Zürich – “Quantum agents who reason in paradoxical scenarios”
- Mike DeWeese, UC Berkeley – “Geodesic optimization in thermodynamic control“
Featured below are research highlights as discussed in several of the speakers’ presentations:
From Josh Deutsch, UC Santa Cruz – “How to make a computer out of junk DNA“:

Josh Deutsch
This talk is about the relationship between evolution of a cells genomics and artificial intelligence models, and how we could be missing a crucial component of the cell’s intelligence by the way biological techniques compartmentalize our understanding of the cell’s components.
Recently there has been a lot of work revisiting the question of the role of so-called “junk DNA” in biology. It turns out that only about 3% of our genome codes for proteins. It was hypothesized that the rest of it – the “junk DNA” – was the result of infiltration by viruses and other unfortunate mechanisms, and it was thought to have no purpose other than perpetuate its own existence.[1]
I show that there is a simple mechanism that transforms this junk into something capable of doing collective computations that closely resembles the neural network models used to do the amazing things that we see today in artificial intelligence applications. I was able to figure out a mathematical mapping to get the creation rate as a function of bound and unbound RNA. With this theoretical prediction, I could then simulate a soup of RNA molecules being created and destroyed, and see if indeed this led to the same kind of learning seen in artificial neural networks. You can use such a model as an “associative memory” or as a way of learning input output relations, similar to the “Hopfield Model” or “Boltzmann Machines“.
These models have a lot of interesting features not present in either conventional computer circuitry or conventional genetic regulatory networks. For one thing, you can mutate these network connections quite a lot, and it doesn’t make much difference to their function. This means they’re quite robust to noise, or evolutionary changes, so you would expect to see far more mutations in these kinds of networks and they would probably appear not to be under evolutionary constraint. Yet they would be doing a great deal of sophisticated computation.
Learn more about this research here.
From Mike DeWeese, UC Berkeley – “Geodesic optimization in thermodynamic control“:

Mike DeWeese
Utilizing a geometry-based technique developed previously by David Sivak and Gavin Crooks.[2][3] Patrick Zulkowski and Mike DeWeese have been able to compute the optimal protocols for performing various operations on nanoscale devices, such as erasing a bit of information from a memory storage device subject to thermodynamic fluctuations. Achieving erasure in finite time necessarily drives the system out of equilibrium, requiring this new framework that can go beyond the more traditional equilibrium approaches. Since different protocols cost different amounts of work, this is an important step towards understanding and ultimately designing nanoscale computational components that can operate efficiently near the limits imposed by physical law.
The authors have applied this approach to several outstanding problems relevant to future computing technology. In one paper they computed the optimal timecourse for lowering and raising a barrier between two wells, as well as that of the relative depth of the two wells, in order to erase a bit of information stored in the location of a colloidal particle subject to thermal fluctuations. They were able to obtain a simple closed-form mathematical expression for the minimum amount of energy that must be delivered to the system in the form of useful work that must be ultimately lost to the heat bath in order to perform this erasure in a finite time period. The study provides clear predictions for future experiments that can be performed using table top apparatus involving a bead in a fluid subject to spatially dependent forces from a laser.
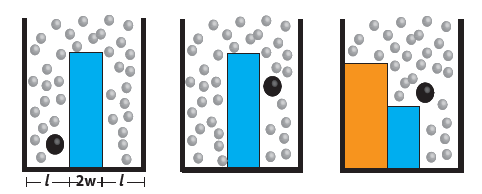
Optimal bit erasure
These studies are important first steps towards the development of nanoscale components needed for the next generation of computational devices capable of overcoming, and even exploiting, the thermal environment.
You can learn more about this research here.
From Lee Alternberg, University of Hawaiʻi at Mānoa – “How much information can natural selection maintain?“:

Lee Altenberg
For the first 20 years after Manfred Eigen published his ideas about the “error threshold” and “error catastrophe“–limits on the amount of information that natural selection could maintain in the face of noisy replication–these ideas never received more than 15 citations a year. But since the 1990s the ideas literally “went viral” when sequencing of viruses revealed the relevance of Eigen’s ideas. Since 2010 the ideas are cited in over 300 papers a year and climbing. But several researchers (Hermisson et al. 2002, Bull et al 2005, Tejero et al. 2011, Schuster 2013, Schuster 2015), have pointed out that the “error catastrophe” is an artifact of Eigen’s needle-in-a-haystack fitness model, and that more realistic models of fitness landscapes do not show a complete loss of genetic information above a threshold mutation rate.
Here I apply a measure introduced by Strelioff et al. (2010) for how much information natural selection is injecting into a population to two models of natural selection: the classical multiplicative selection model in which fitness decreases exponentially with the number of mutant loci, and a “quasispecies yo-yo” model, constructed to exhibit four error thresholds in which the genotypes switch back and forth between mostly “0” bases and mostly “1” bases. The multiplicative model can be solved exactly, and reveals that the genetic information maintained in the population decreases gradually with increasing mutation rates. A similar outcome is found for the “yo-yo” model except that there is an extremely narrow range of mutation rates around the error thresholds where the genetic information drops, where the two competing quasispecies are nearly equal, and immediately rises again for higher mutation rates.
What we see in these results is that mutation rates can put a limit on the amount of information per site maintained by natural selection, but this does not limit the total information maintained, and longer and longer sequences can maintain more and more genetic information.
You can learn more about this research here.
Learn more about the Manoa Mini-Symposium on Physics of Adaptive Computation on the symposium website. For more information about the Thermodynamic Computing workshop check out the recap blog.
[1] The ENCODE project examined what this DNA does and found that about 80% is transcribed and shows biochemical activity, however only about 5% of the genome can be identified as being under evolutionary constraint. This apparent contradiction is still the subject of much debate between those that maintain that this DNA is just inadvertently being transcribed to RNA, and those that believe that many recent works has shown specific biochemical pathways that junk DNA is involved in. There is however a large body of work finding specific functions of such non-coding RNA, but the amount that is clearly functional is still very much up in the air.
[2] https://arxiv.org/abs/1208.4553
[3] https://arxiv.org/abs/1201.4166